The Network Science PhD program at Northeastern University is a pioneering interdisciplinary program that provides the tools and concepts aimed at understanding the structure and dynamics of networks arising from the interplay of human behavior, socio-technical infrastructures, information diffusion and biological agents.
The PhD in Network Science is an interdisciplinary program supported jointly by the Khoury College of Computer Sciences, College of Social Sciences and Humanities, College of Science, Bouvé College of Health Sciences, D’Amore-McKim School of Business, and College of Arts, Media and Design. Our interdisciplinary program provides the conceptual and mathematical tools needed to describe and predict networks in an ever-evolving field. You’ll learn a range of network-based approaches, and will become proficient in relational (graph-based), mathematical, and algorithmic techniques, as well as fundamental network theories that can be used to explain mechanisms and processes of networks across domains—in physical, technological, informational, and social systems.
As the only Network Science program based in the U.S., our curriculum provides a scholarly experience that is truly one of a kind. The program fosters intellectual and quantitative agility, enabling you to combine theoretically-driven inquiries with data collection and analytic tools and theories—while also understanding when, and in what contexts, particular techniques are appropriate and meaningful.
Admitted students typically receive financial support in the form of a Graduate Assistantship for 5 years while in good standing in the program. This support typically includes a stipend, tuition remission, and health insurance. Assistantships are provided for the first five academic years, plus the first summer. Continuation of your Assistantship is contingent upon fulfilling all academic and graduate assistantship requirements and may be facilitated through your pursuit of external funding sources, such as fellowships or grants. Conditions relating to PhD Student funding can be found here.
Tuition Remission
Student support typically includes a tuition waiver for coursework pertaining to studies.
Graduate Assistantships
Stipends are provided through Graduate Assistantships. The responsibilities required for Assistantships vary, but typically come in the form of Fellowships, Research Assistantships or Teaching Assistantships. Assistantships are offered with the expectation that the student devotes 20 hours per week to their assistantship duties. Students are admitted into the program with a faculty advisor. Student’s assistantship duties are assigned and performed under the supervision of their faculty advisor.
The stipend rate Network Science Institute for 2022-23 will be approximately $40,000 per year, including the summer. Students are typically paid on a twice-monthly basis, in the amount $1,673. Stipends are subject to taxes and other mandatory deductions. Assistantships and financial support includes the summer term.
Research Assistantships (RA)
Support is primarily maintained through research assistantships (RA), for which students are expected to devote 20 hours per week to research assigned through the student’s faculty advisor. RA positions give students the opportunity to participate as members of a research team. Frequently this work leads to the doctoral thesis projects. Students should expect to spend additional time conducting research in support of their academic studies, such as coursework and activities connected to the dissertation.
Teaching Assistantships (TA)
Graduate students holding teaching assistant appointments must register as full time resident students and are charged full tuition during the period of appointment. The TA appointment comprises a monthly stipend as well as a tuition scholarship which is credited directly to the student’s account. Teaching Assistants are compensated on the basis of the time devoted to teaching activities. A 100% graduate TA appointment typically includes payment of full tuition for the period of the appointment. Partial appointments (for effort less than 100%) are also possible, and are frequently used to supplement outside fellowships, scholarships, or research assistantships. Students who receive financial support from multiple resources must do so in accordance with department and university guidelines. A 100% teaching assistantship requires no more than 20 hours of effort per week. Northeastern recognizes that graduate students may spend additional time conducting research in support of their academic studies, such as coursework and activities connected to the dissertation.
Other Financial Support
There are also funding opportunities available through external sources where the funds are provided directly to the PhD student rather than through Northeastern University. External doctoral fellowships typically offer stipends comparable to the NU stipend, and some awards offer additional travel or equipment funds. These awards are competitive and rare, though Network Science students have had success with them in the past. If a student receives an extramural award, the external funds will be applied towards stipend and tuition/fees, and the program and/or advisor will supplement as needed to ensure students receive at least the standard support outlined in the admissions letter.
Occasionally students have need for supplemental financial support. Information on financial aid loans can be found here.
Health Insurance
All students in the NS PhD program receive health insurance and tuition remission. While Northeastern University covers the cost of the individual graduate student health insurance plan (NUSHP) for its fellowship students and graduate assistantships, you will be required to pay all other university-issued fees (including student fees, roughly $130 per semester, the University Health and Counseling Service fee, estimated at $225 per year, and an additional one-time fee of approximately $350 for international students). Additional information on the student health plan can be found here.
We welcome applicants from talented students from all countries around the world. If you have any questions about immigration issues, please feel free to contact us. View President Aoun's statement on embracing our global community here.
International students admitted to the Network Science PhD program typically receive an F-1 Visa. The Office of Global Services is available to answer questions regarding international student The following documents are typically required for the F-1 visa application:
Online Non-immigrant Visa Application Form (DS-160) confirmation page
Visa application fee payment receipt (visit the U.S. Embassy or U.S. Consulate website for specific instructions)
Form I-20 issued by OGS at Northeastern University
Valid passport (the passport should be valid for at least six months after your date of entry into the U.S.)
Evidence of financial support (proof of sufficient funds for estimated cost of one academic year)
Student and Exchange Visitor Information System (SEVIS) I-901 fee payment receipt
A copy of your acceptance letter to Northeastern University
One photograph (see the U.S. Department of State website for photo requirements)
Our Network Science doctoral program receives applications from students around the world with diverse backgrounds and research interests. The backgrounds of our incoming students are quite diverse, ranging from mathematics, physics, and computer science, to biology, to epidemiology to psychology, political science, communication, and other social sciences.
The application deadline for Fall 2024 is December 1, 2023.
International Applicants
We welcome applicants from talented students from all countries around the world. If you have any questions about immigration issues, please feel free to contact us. View President Aoun's statement on embracing our global community here.
Admission Requirements
A minimum of a baccalaureate from an accredited institution is required. Proficiency in the English language is essential. Students selected must meet minimum requirements for admission to the University (overall undergraduate GPA of 3.0, and a combined GRE Verbal + Quantitative score of 297). Note GRE is optional for applications this year. However, a GPA of 3.5 and a combined GRE V/Q score of 311 are considered competitive.
International students must submit TOEFL, IELTS, or Duolingo scores unless they have earned a degree at an institution where English is the medium of instruction. Students must receive a minimum score of 100 on the Internet-based TOEFL. The TOEFL and GRE scores should be sent to the College of Science. The institution code for College of Science is 3682.
The following material must be included as part of your application:
Application and application fee*
Transcripts from all schools attended
GRE scores are recommended
Proof of English proficiency (TOEFL exam, IELTS exam, Duolingo exam, or a degree earned at an institution where English is the medium of instruction).
Three letters of reference (two of which must address academic competencies)
Resume
A statement of interest (1-2 pages)**
* The application fee for both domestic and international students is $95. After completing the on-line application, directions will be provided for the payment. *If this is a financial burden, on a case by case basis, we will offer application fee waivers.
**The statement of interest (SOI) should include a description of your interest in the program and your career goals Your essay should demonstrate knowledge about the program, and of the types of research carried out by Network Science Institute faculty, and how you and your interests fit with that. It should also include the ways in which your experiences and/or coursework have influenced your development, interest, and your potential as a scholar.
Applicants should indicate interest in one of the network science institute faculty and/or core research areas as part of their application. Faculty research, lab groups, and recent publications can be found within the Institute website.
The program takes a mentoring approach whereby the graduate students work in research groups of faculty, doctoral students, and postdoctoral fellows, with opportunities to conduct research across traditional disciples utilizing network methodologies. In the laboratory, responsibility for collaboration in research gradually shifts from the faculty advisor to the student, culminating in the student’s doctoral dissertation.
Each student will have one primary faculty advisor from the Network Science Doctoral Program faculty. Students are admitted into the program with a faculty advisor. Students will be expected to select their dissertation advisors by the end of the spring semester of their second year in the program. In most cases, the student’s faculty advisor is expected to become their dissertation advisor.
Applications should be submitted through the SLATE application portal: https://enroll.northeastern.edu/apply/
For advice on applying for a Ph.D., see:
“Graduate School Q&A” by Brooke Foucault Welles
“Everything that you wanted to ask about applying for grad school but were afraid to ask” by David Lazer
For other good advice on applying to graduate school see
“Top 10 Tips for Writing a PhD Statement of Purpose”
“Applying to a Ph.D. Program”
The priority application deadline for Fall 2024 is December 1, 2023.
The following material must be included as part of your application:
· Personal Statement
· Transcripts from all institutions attended
· 3 letters of recommendation
· GRE scores recommended but not required
· Proof of English proficiency (TOEFL exam, IELTS exam, Duolingo exam, or a degree earned at an institution where English is the medium of instruction).
Admission for the Network Science program is housed in the College of Social Science and Humanities. For the application, be sure to select [Social Science and Humanities] in the [College/School] field. Network Science should then be listed as a program.
Please contact the Graduate Student Service office (gradcssh@northeastern.edu) to check if your transcripts and test scores have been received. The Graduate Student Service office receives and processes all application material for the Network Science PhD program. Please note that an electronic copy of your transcripts, uploaded with your application, are required for the review process. Should you be accepted, and decide to enroll, an official copy of your transcripts is required within 30 days of matriculation.
As with many PhD programs, admittance to the Network Science PhD program is determined on a case by case basis, where applicants’ background and area of academic interest are evaluated by the faculty who sit on the admission committee, and each have their own criteria. It is difficult to put forth a metric or guideline as to what they are specifically looking for. In past admission cycles, there was a high number of applications for a limited number of seats, and the admission committee was selective. Of the students who were admitted, the average GPA was over 3.5 on a 4 point scale. GRE Qualitative, Quantitative, and Analytical Writing scores were in the upper percentiles.
We are happy to provide applying students with an application fee waiver. On the Application Information page, under the Application Fee Waiver Code field, please enter “GOCSSH” without the quotes and you should be able to submit your application without needing to pay the fee.
Here are some examples where you may not have to provide us with a language proficiency test score report, but please do be advised that language proficiency will be determined during the application review process. Please note that we consider graduate certificates as a non-degree and as such will not satisfy the language proficiency requirement.
· You have/will receive a bachelor’s, master’s or doctoral degree from a U.S. institution.
· You are a citizen of a country where English is the official/primary language.
· You have/will receive a bachelor’s, master’s or doctoral degree from a country where English is the official/primary language.
· You have have/will receive a bachelor’s, master’s or doctoral degree abroad from an accredited, American university abroad.
· English is the medium language of instruction where you have/will receive a bachelor’s, master’s or doctoral degree. This information must be clearly stated on your transcript.
The TOEFL and GRE scores should be sent to the College of Social Science and Humanities. The institution code for College of Social Science and Humanities is 3682.
As we continually look for ways to improve and enhance the student experience, Northeastern University has recently moved to Slate, an admissions application platform designed to provide each student a unique and personalized admissions experience. During this period of transition, you may experience delays in response to your questions regarding application status and updates. For the most up to date information regarding the standing of your application, please visit enroll.northeastern.edu/apply/status
For questions unrelated to Fall 2023 applications, please allow 1-3 business days for a response.
The financial support typically involves a stipend, tuition waiver, and health insurance; for a total award of approximately $63,000. For the current year, the stipend is $13,390 per semester, or $40,171 for the fall, spring, and summer term. This award amount is subject to change for 2023. Obligations can include both teaching and/or research responsibilities. This determination is not made until after students are accepted, and is contingent on both the student’s background and the current needs of the program.
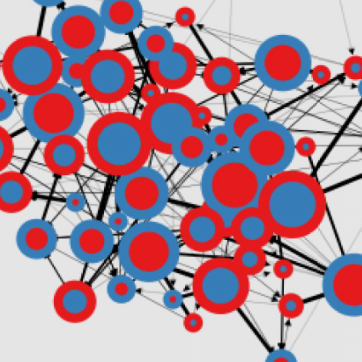
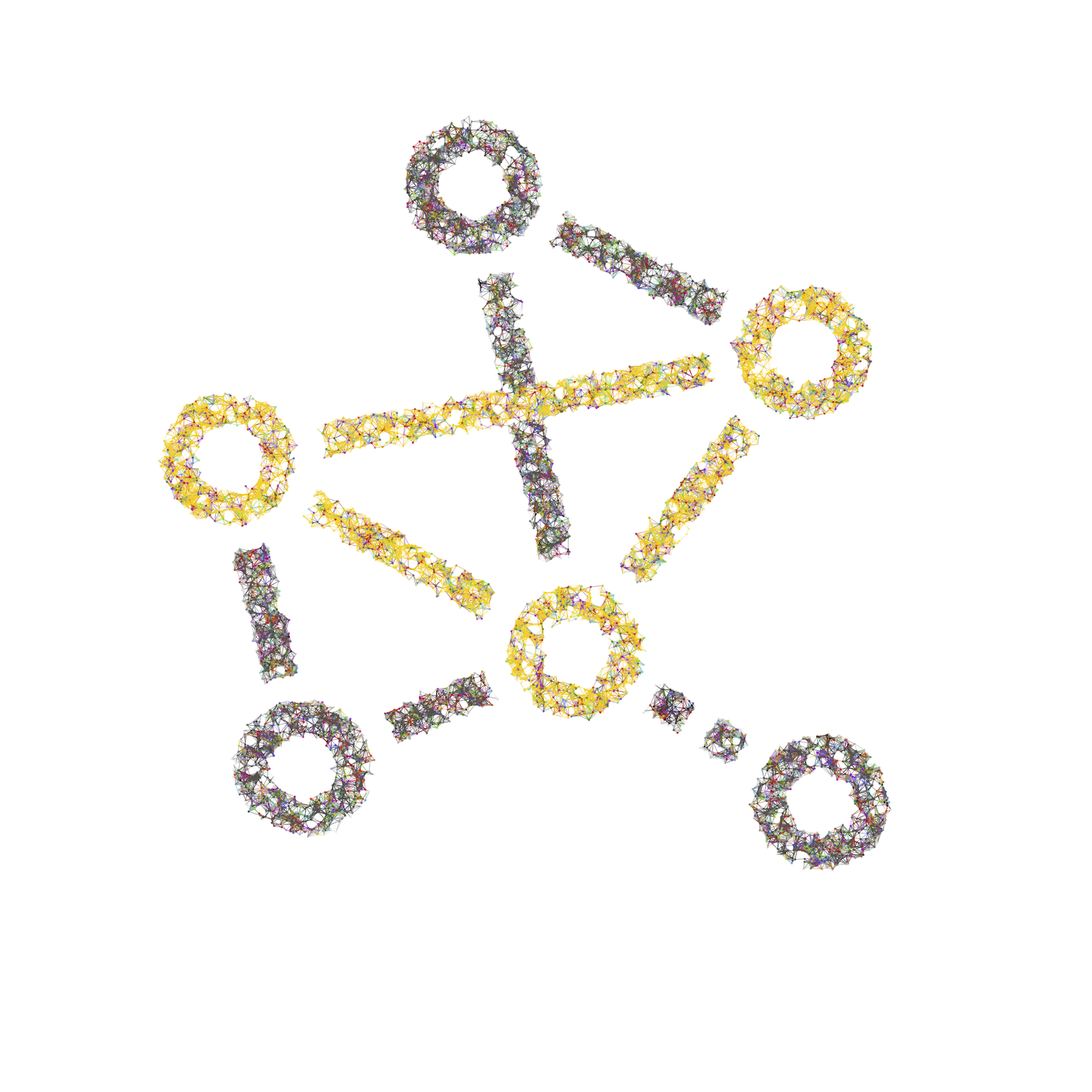
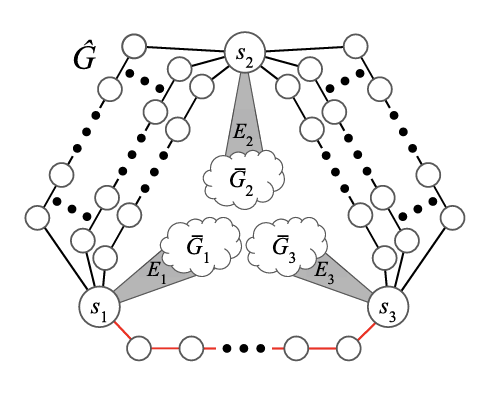
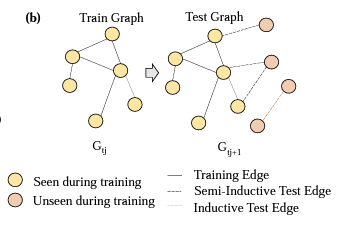
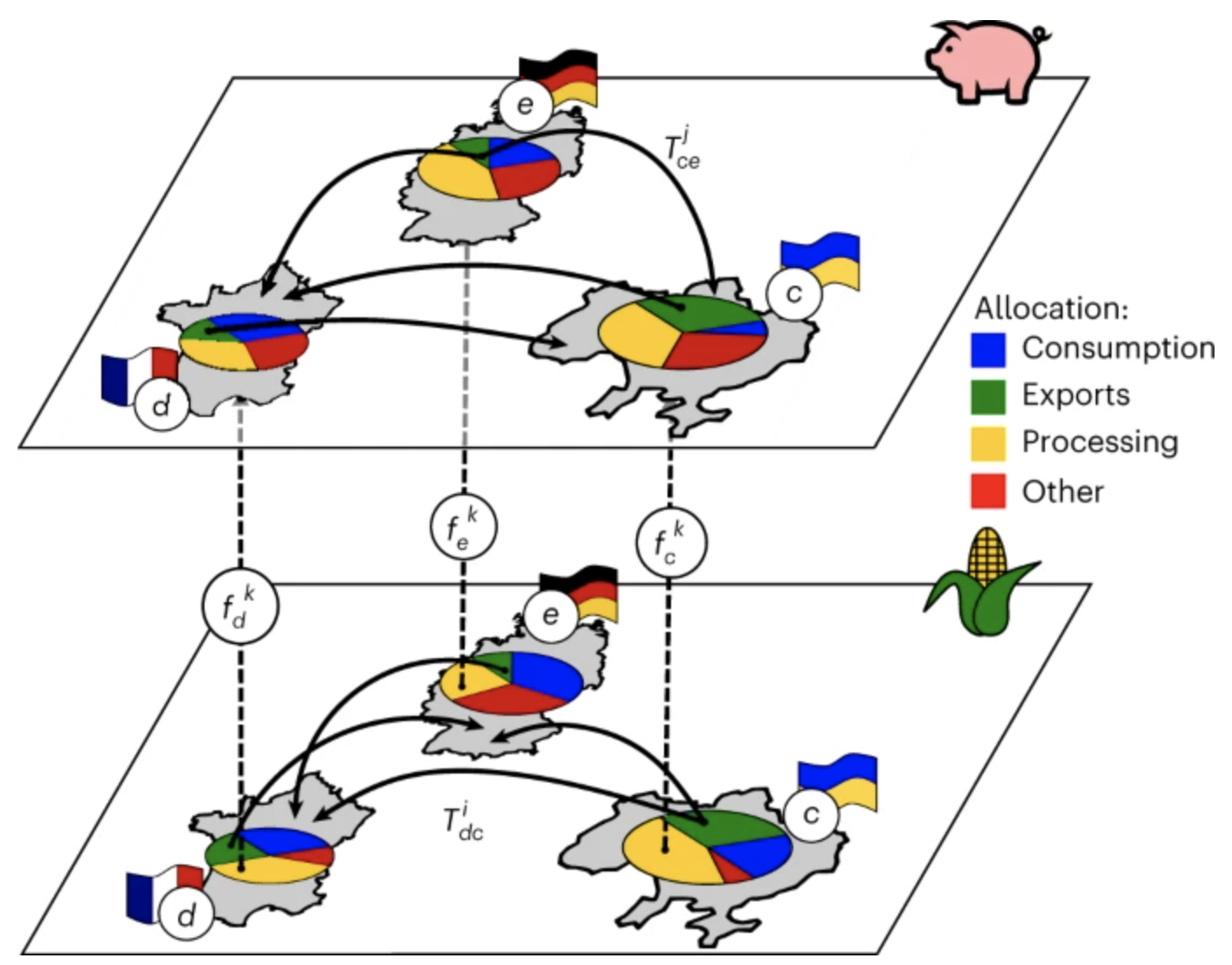
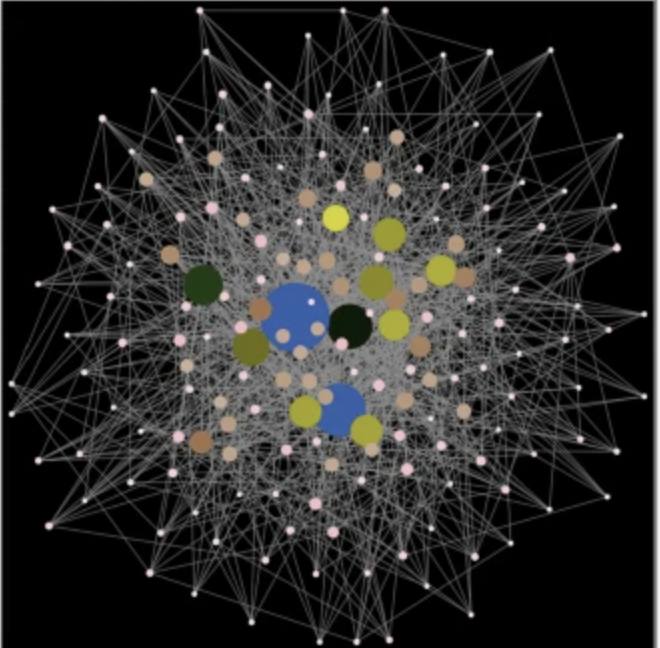
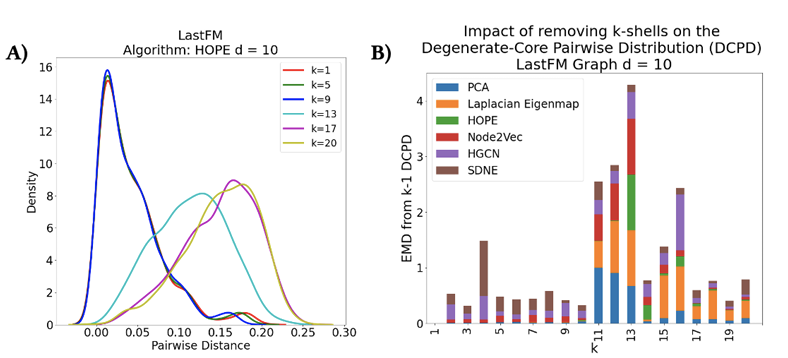

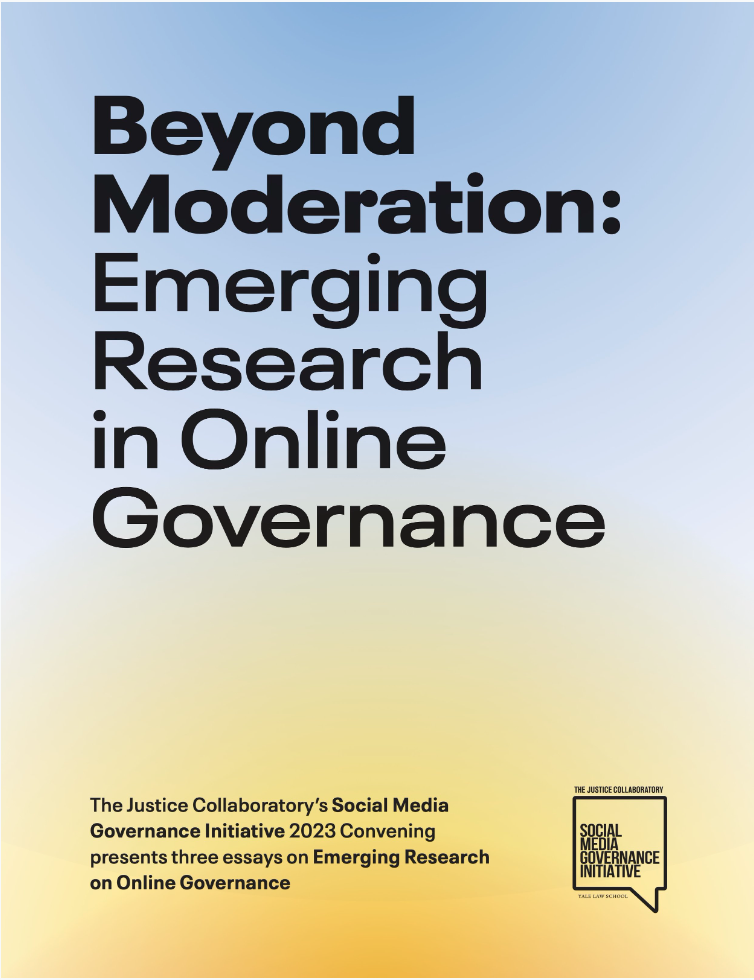
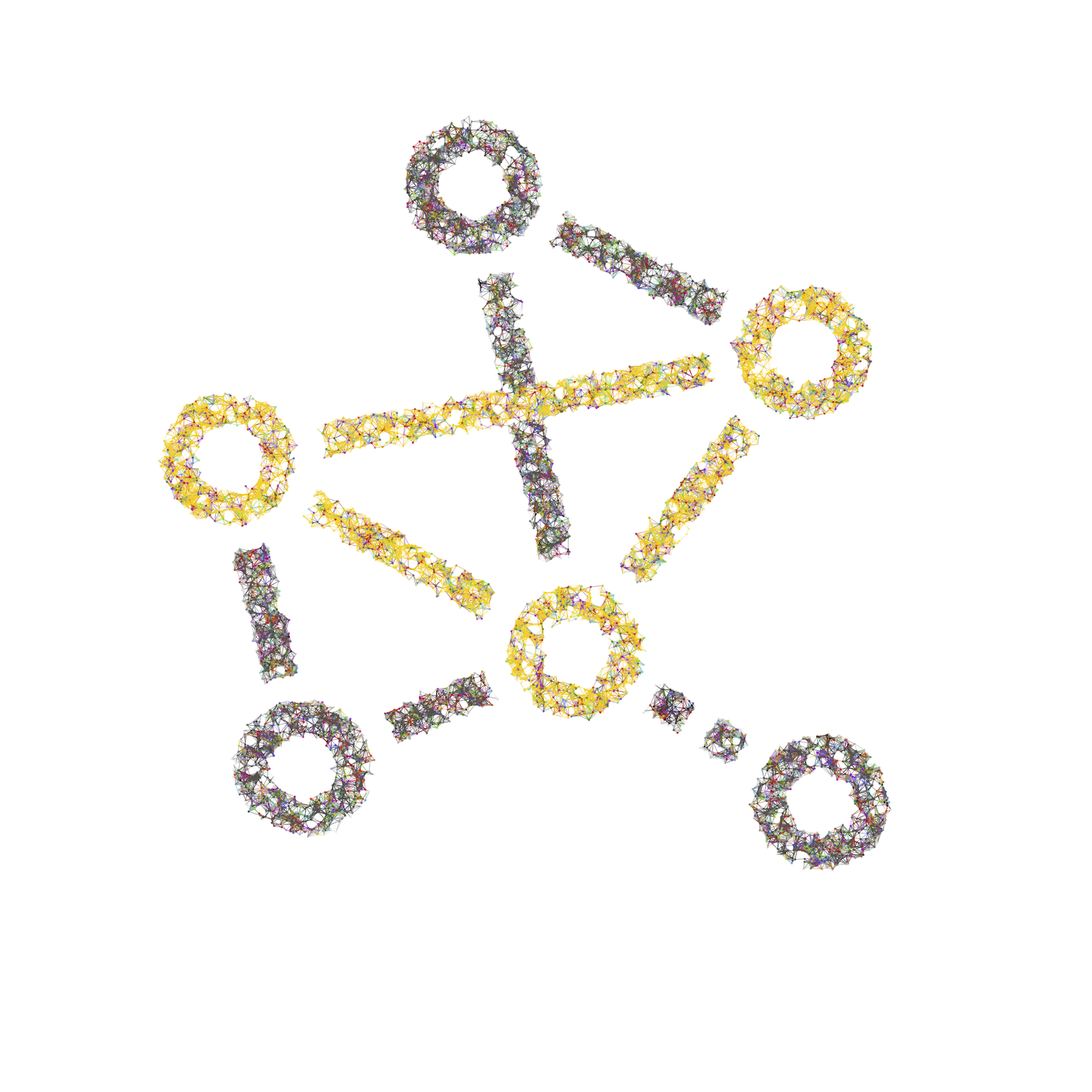
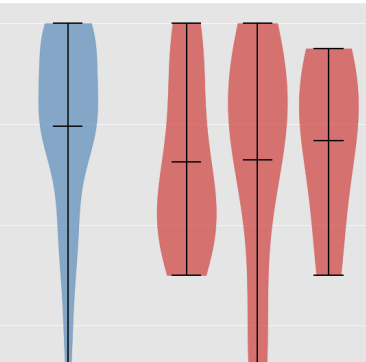


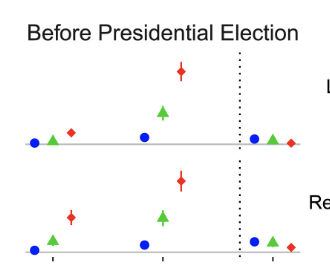
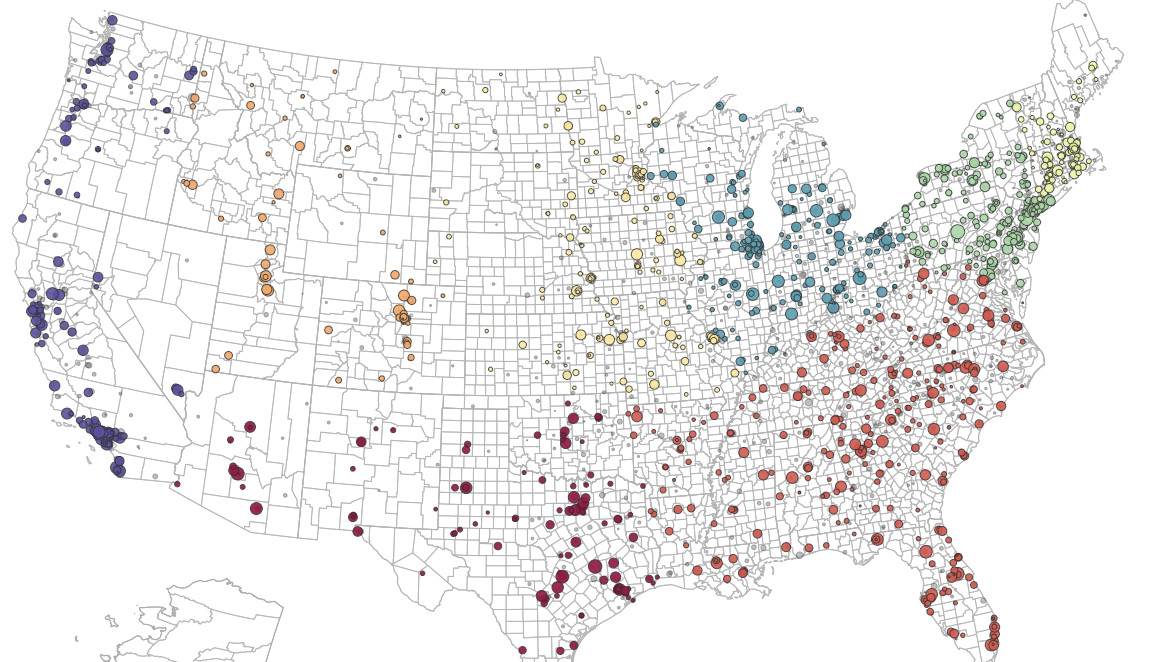
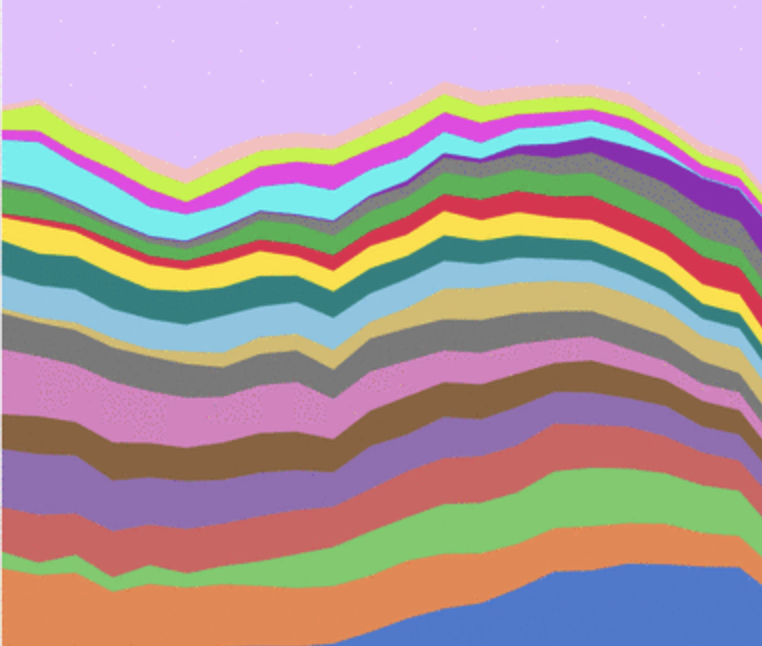
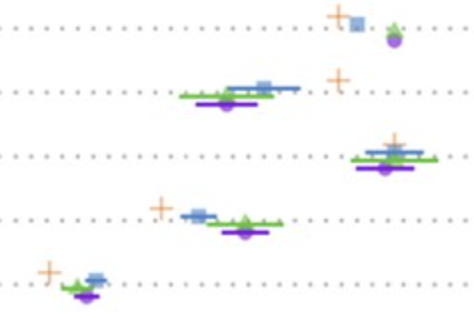
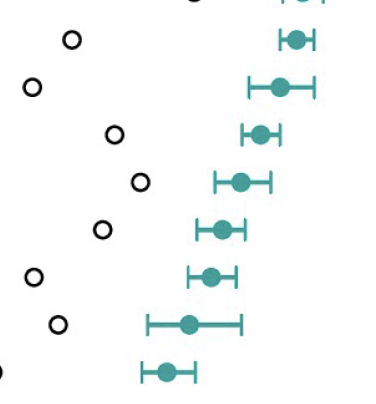
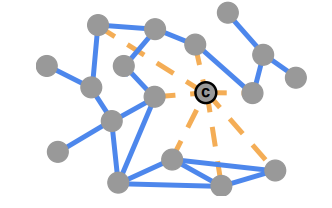
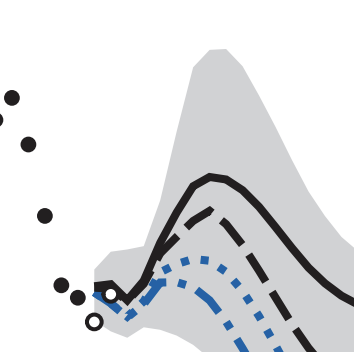
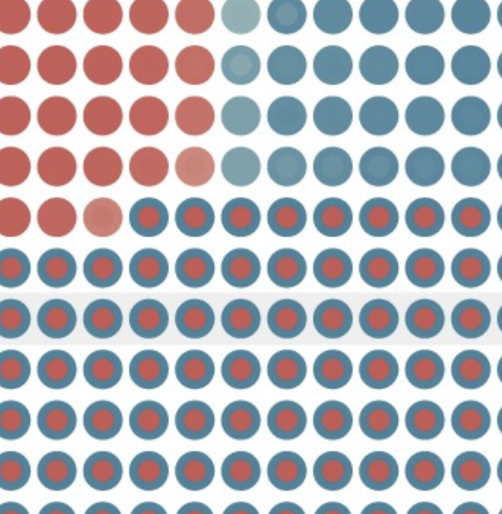
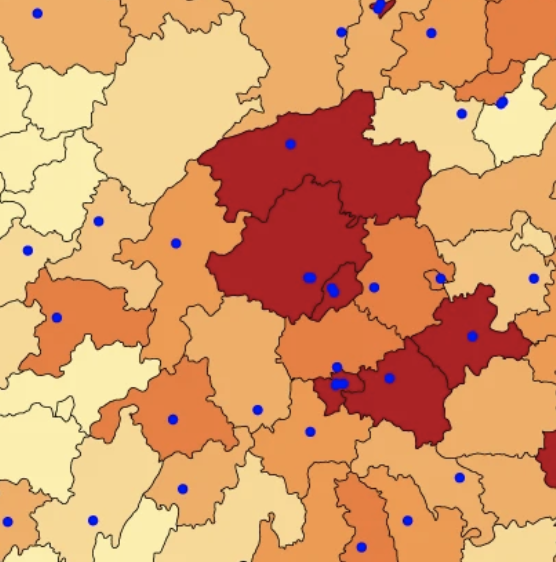
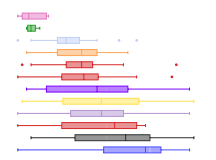
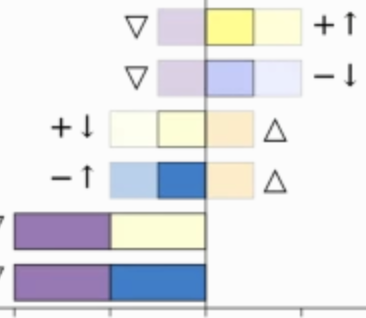
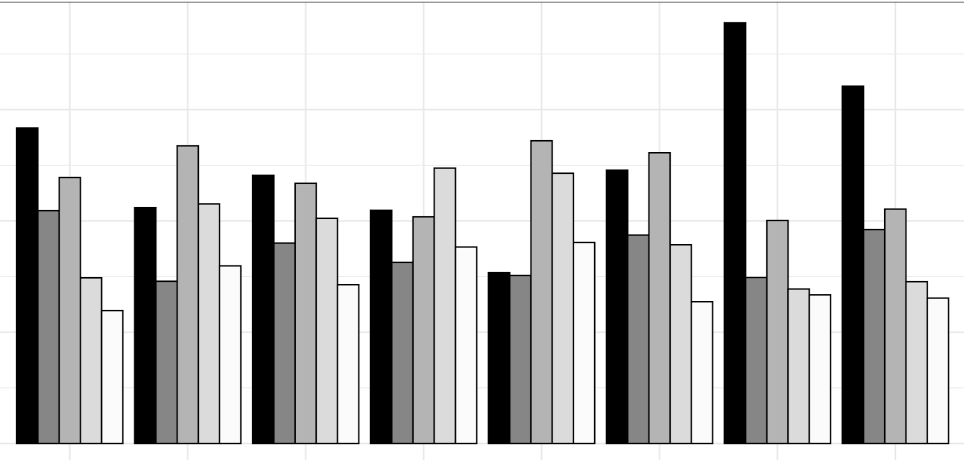
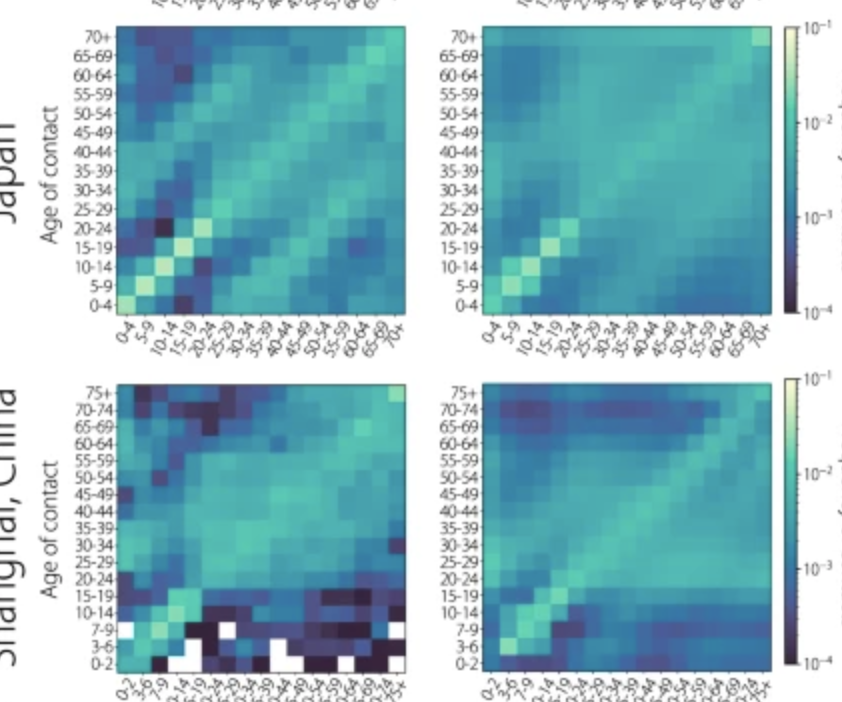
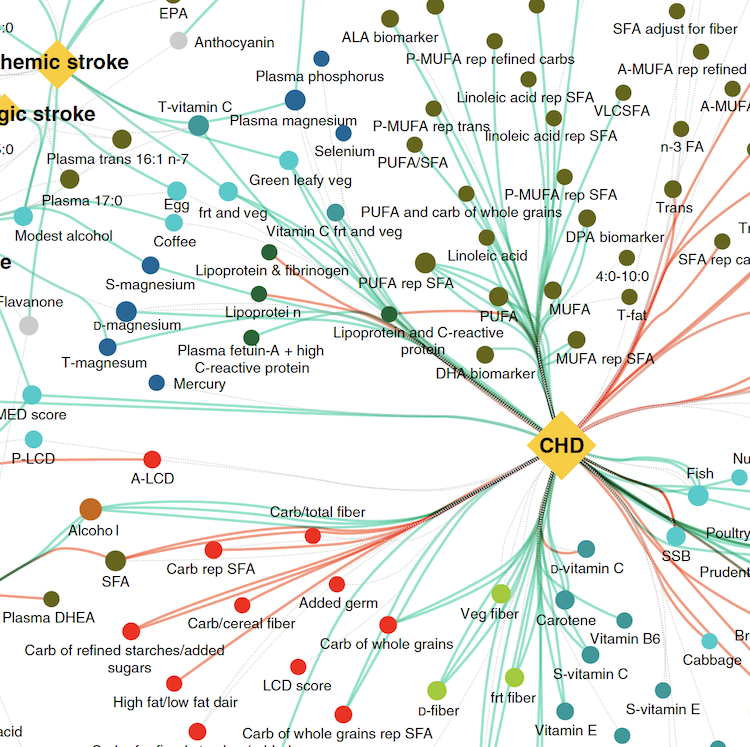
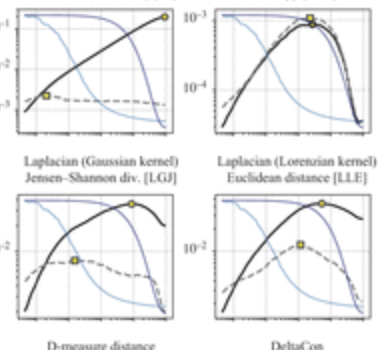
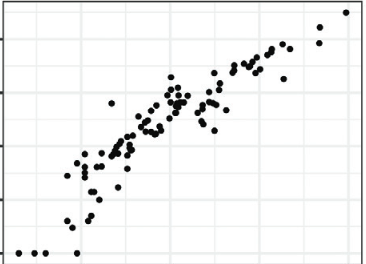
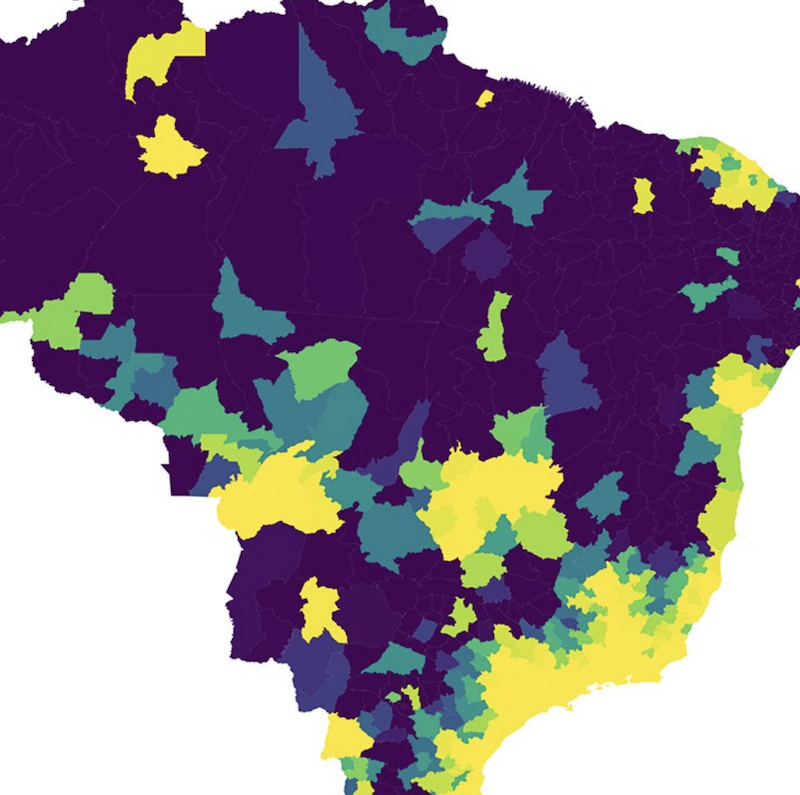
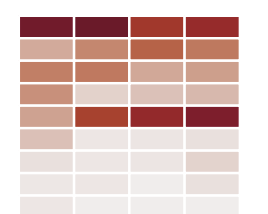
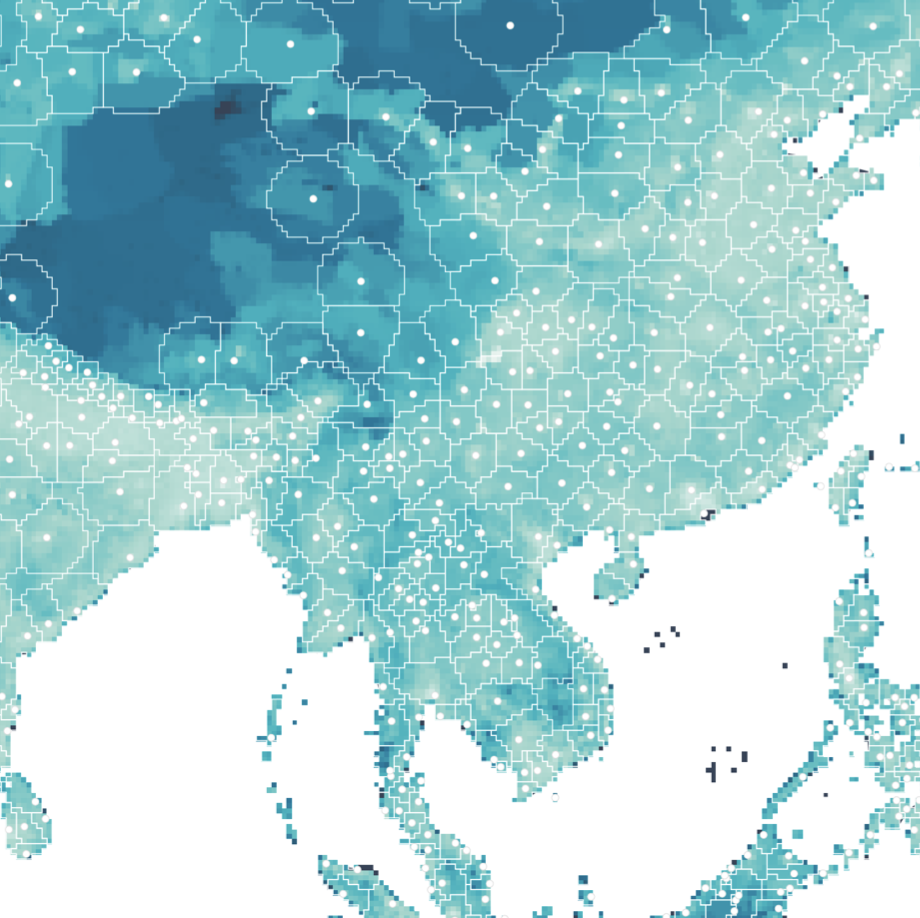
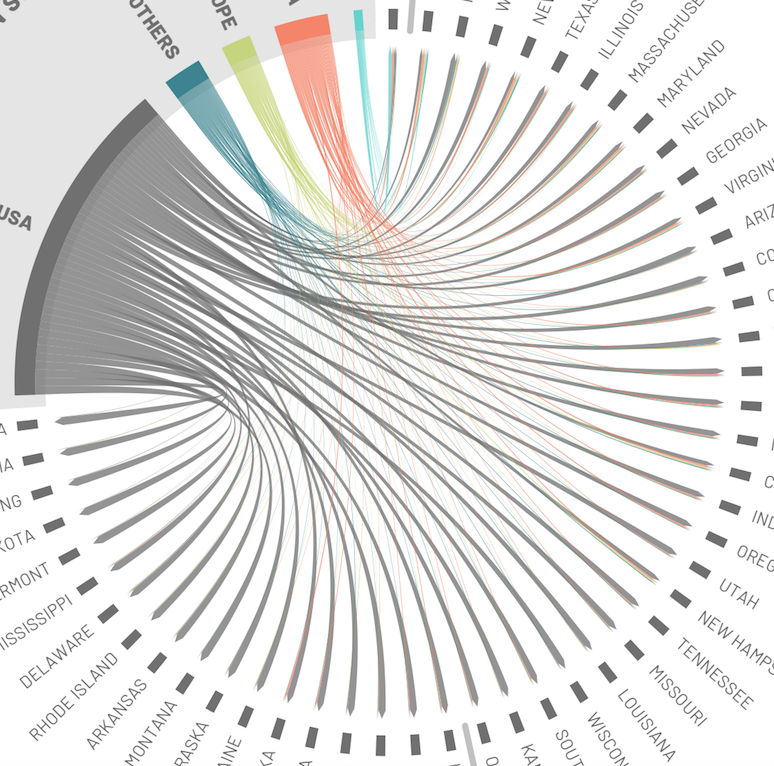
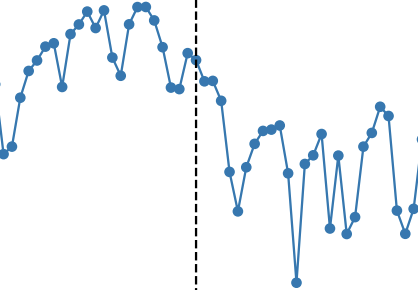
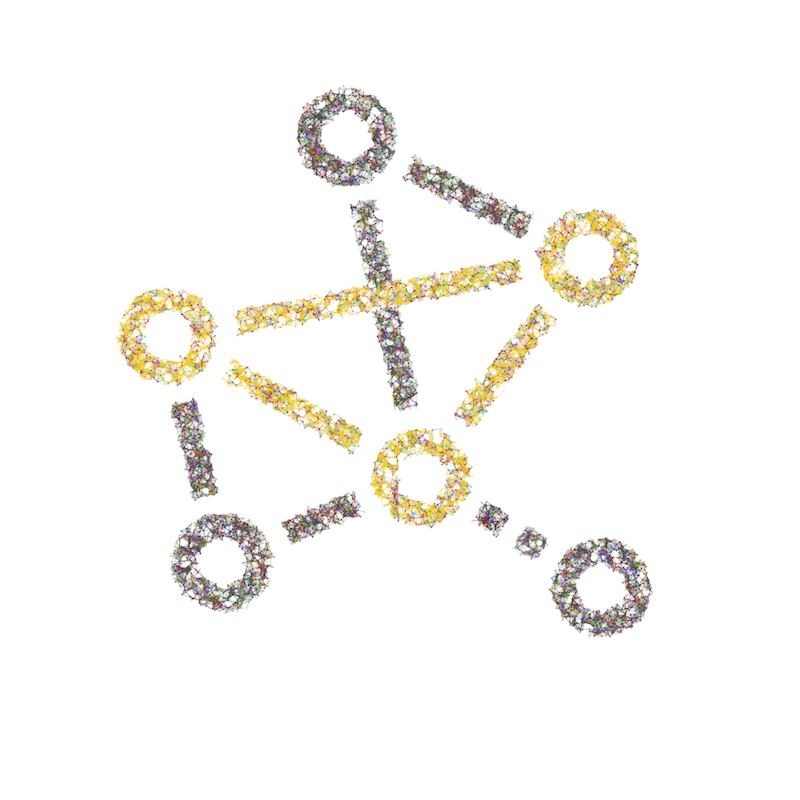
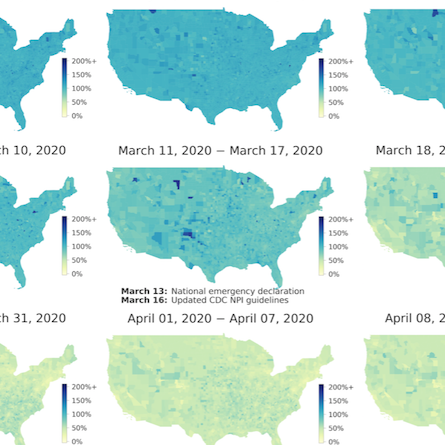
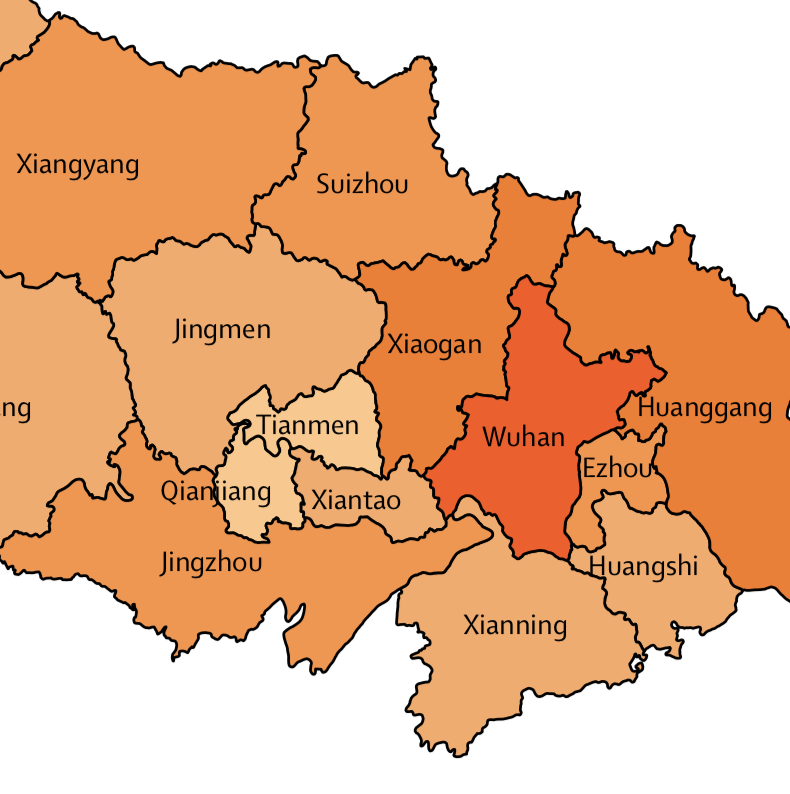
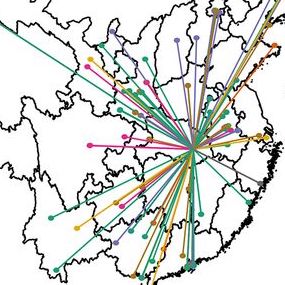
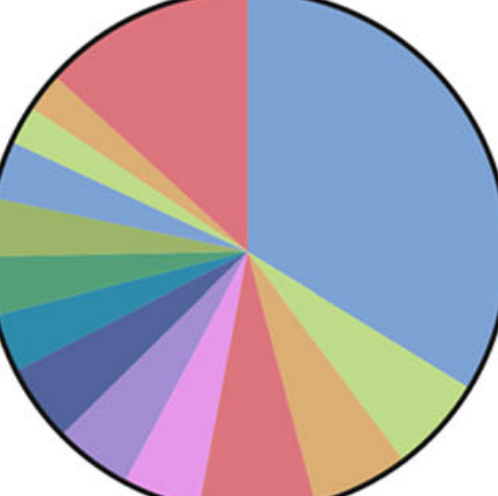
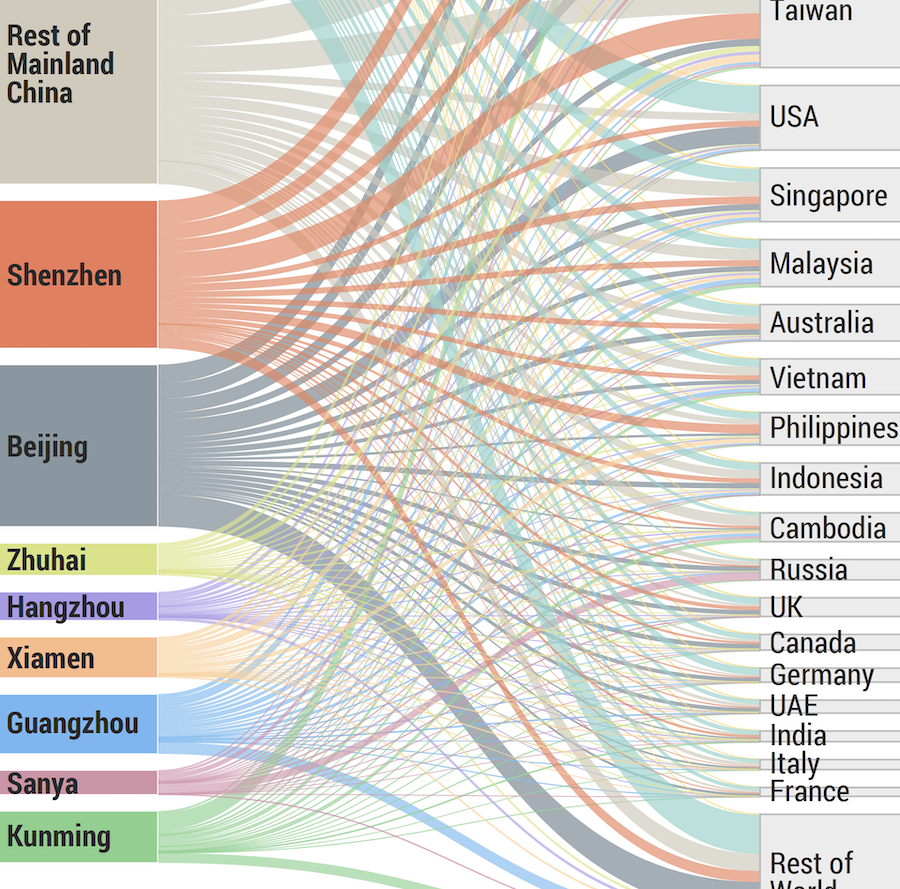
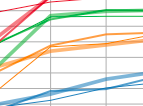
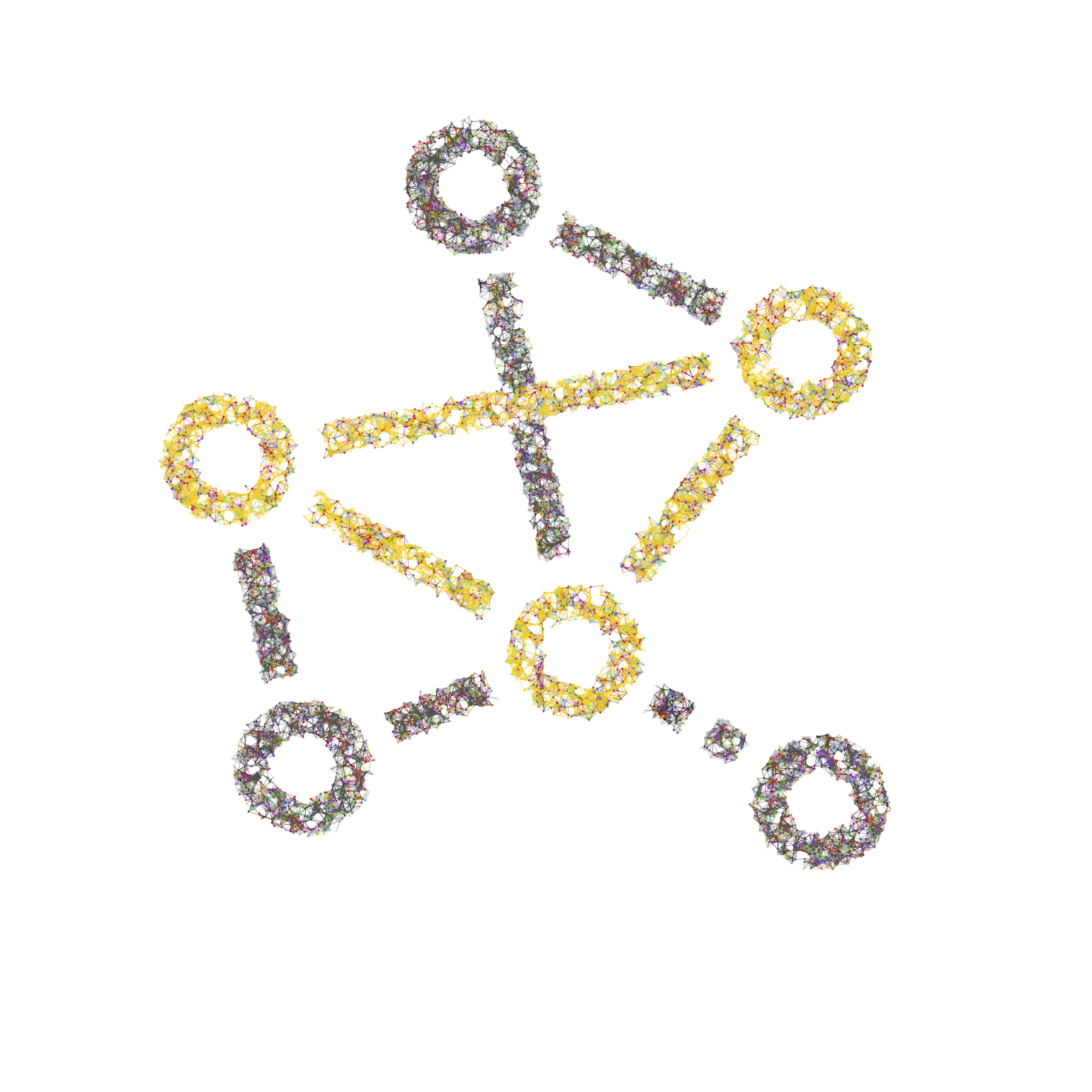
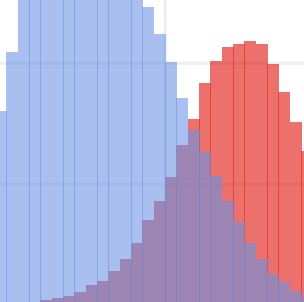
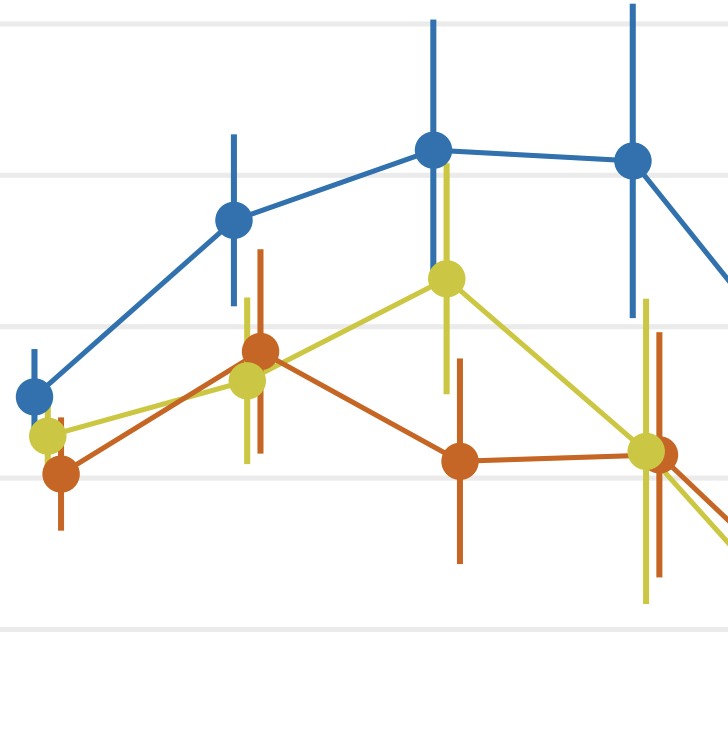
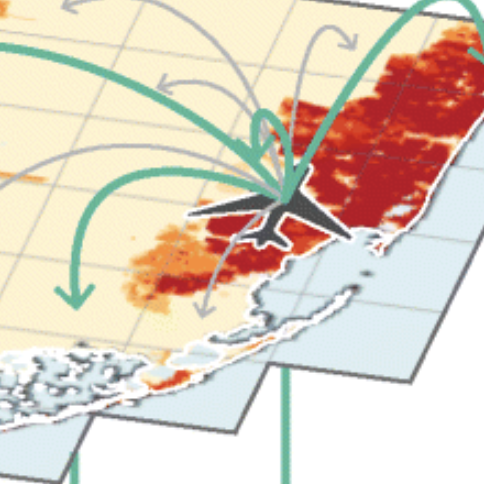
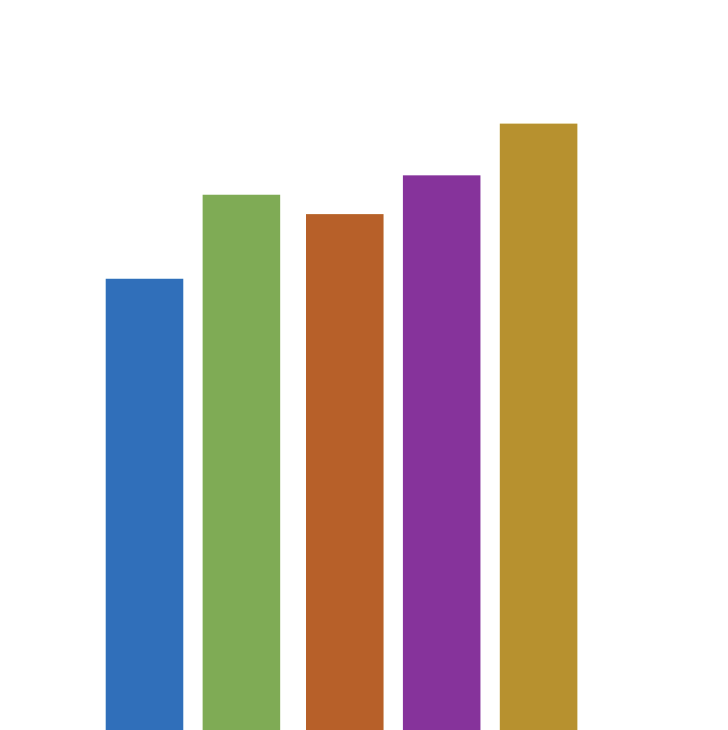
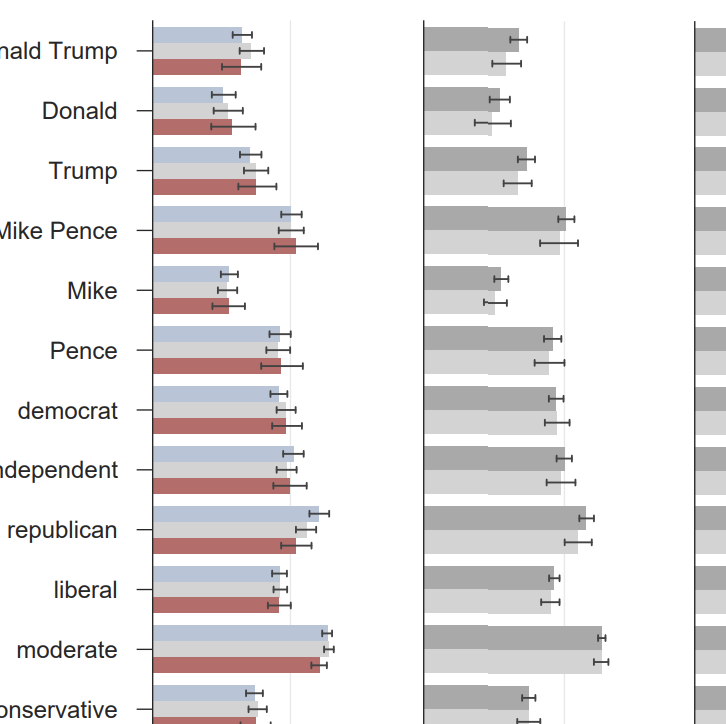
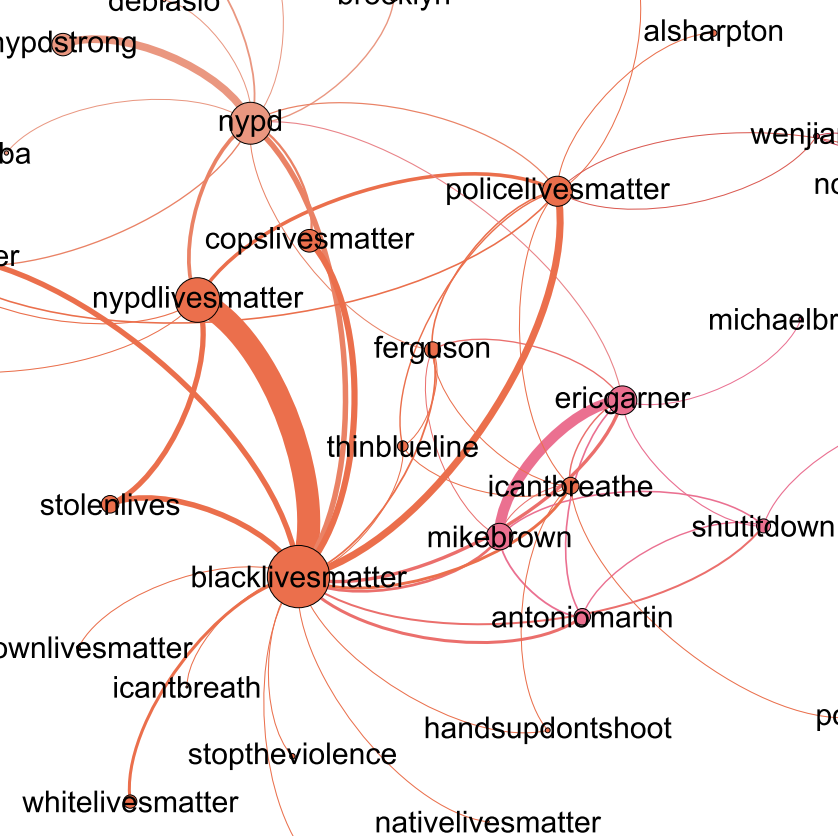
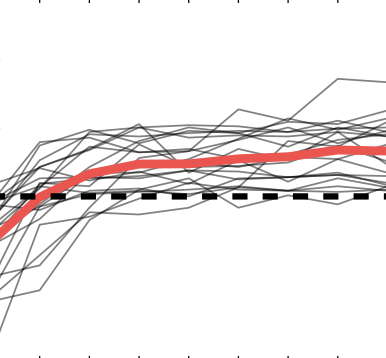
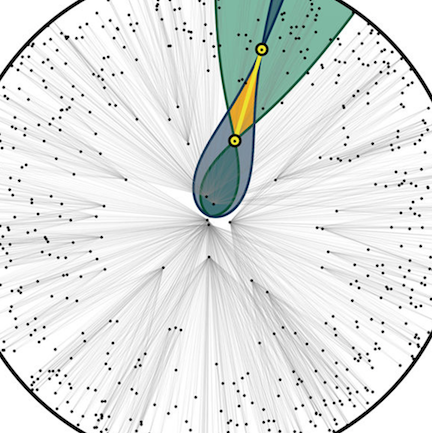
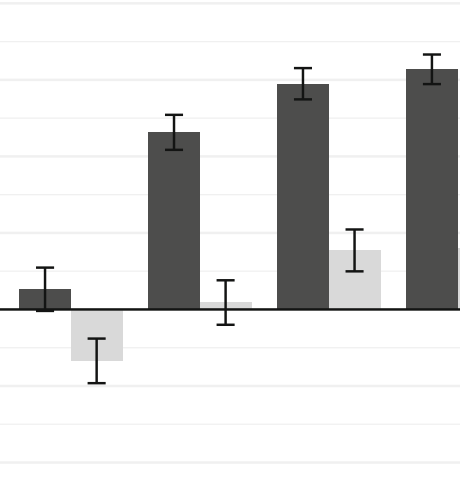
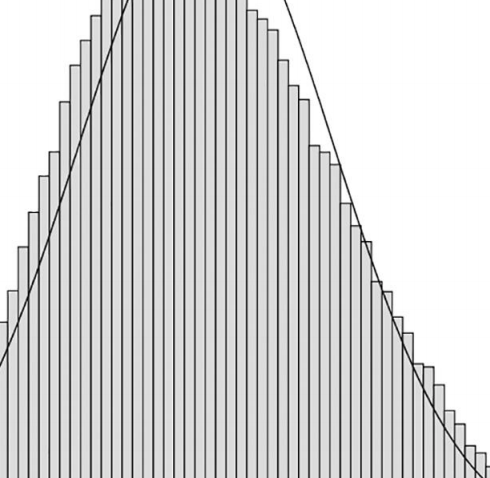
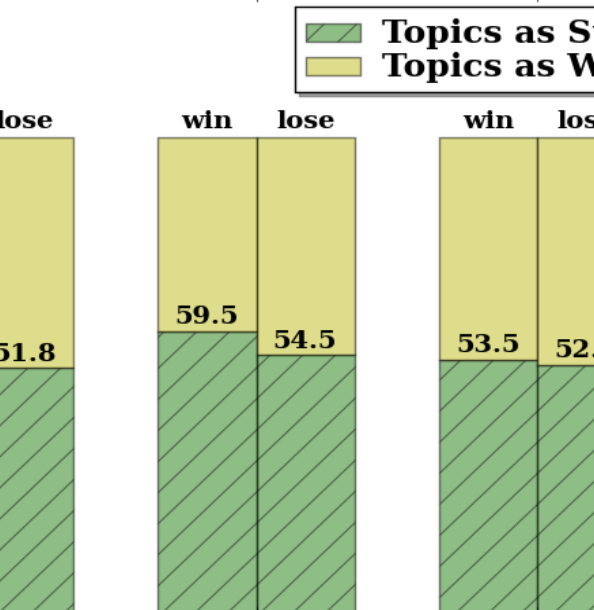
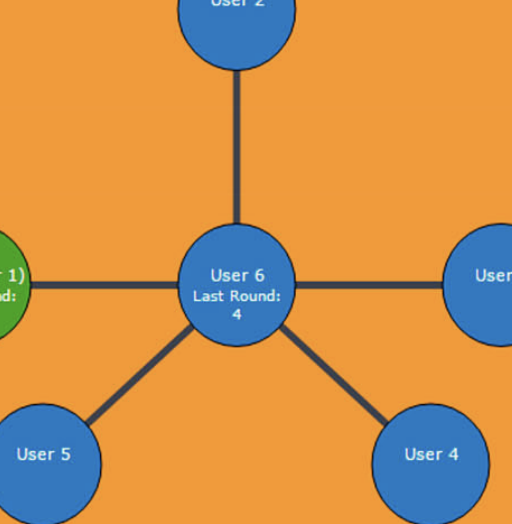
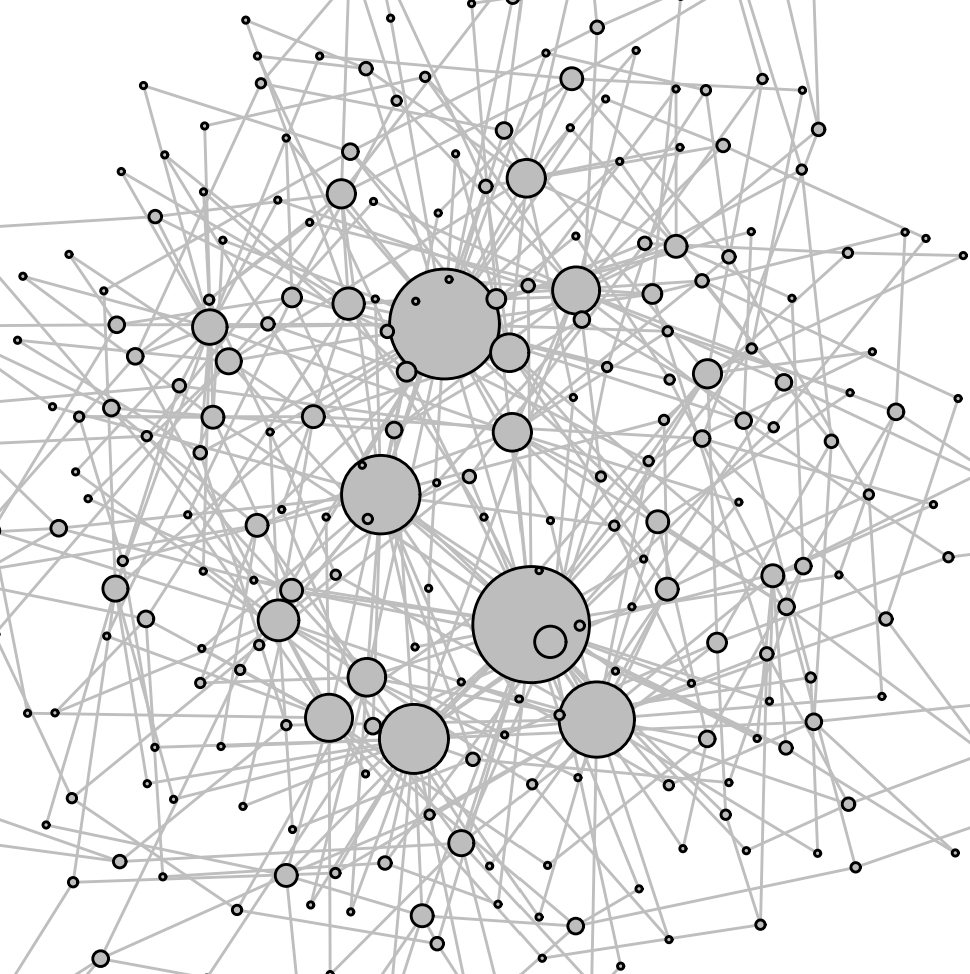
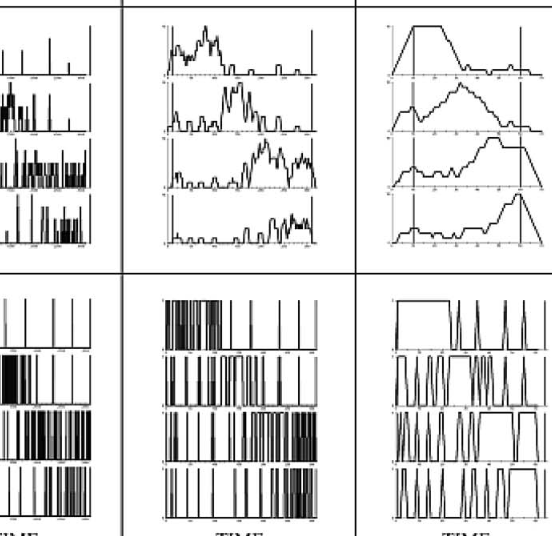